In the following article, we will get the answer on how IoT app development and machine learning are changing the industry outcomes. Let us look on the following details.
When you talk about machines or manufacturing, maintenance comes buzzing around. Whether you buy a car, a household utility, or whether it is any machine at the manufacturing unit. Moreover, everything requires maintenance., the reason is all the machines. No matter their place of use, can break in the long run. In fact, a study by Wall Street Journal & Emerson found that industrial manufacturers have faced unplanned downtime. Moreover, 42% of the time it is due to equipment failure.
What can be done to prevent this substantial loss?
The answer to this is quite simple- engage in an effective machine maintenance strategy.
During the early days, machines were not too complex and hence there were limited breakdowns. However, with the advancements in machines through program and logic controllers the scenario of fewer breakdowns has changed. Previously, there was more maintenance through manual labor contrary to what it is now. The manufacturing units and factories want to stay competitive through rapid assembly lines. Also, tremendous automation through complex machines, thus hiring big data analytics companies. This assists in measuring performance metrics such as production efficiency, output, and equipment efficiency. Because of all this, the “maintenance” was done only during equipment failure. Moreover, it has now become a routine scheduled activity known as Preventive Maintenance.
Understanding the Difference –“Preventive Vs Predictive” Maintenance
However, many are confused between Preventive and Predictive Maintenance. Let us understand the difference with an example. You bought a car and the car manual says you need to change the oil every 1000 km or in 2 months. You then take your car to the shop for an oil change so that your car stays in good condition. This is Preventive maintenance.
Now, this time you bought a car well equipped with all the indicating devices. Also, air filters and all the accessories it can have. You drive this car for 500 km. Then the alert comes in the car that you have 500 km left to change the oil in the car. This is Predictive Maintenance. Such maintenance prevents machines from breaking down and gives a prior alert to repair before the machine actually fails.
Preventive maintenance has been very popular with manufacturing industries so far.
But as a matter of fact, predictive maintenance delivers a different value to the industry. Preventive maintenance is planning and scheduling like in the case of a car. In actuality, your machine does not require fixes based on its current state. Rather it is done through scheduling it, you are bound to go for maintenance. On the contrary, predictive maintenance is based on the actual condition of the machine instead of the scheduled time. This allows companies to predict equipment failures before they occur and provides enough time to schedule future maintenance.
The 4th wave Industrial Revolution has changed the way maintenance has been functioning. With the introduction of technologies Internet 4.0 or the Industrial Internet Of Things. Moreover, the equipment now connects with sensors that utilize advanced analytics and machine learning to draw significant comprehension. Furthermore, these data insights help in scheduling maintenance. This maintenance strategy utilizing machine learning analytics is Predictive Maintenance. Furthermore, this results in substantial savings for companies.
Anomaly Detection in Predictive Maintenance
With machine learning and IIoT gearing up in the manufacturing industry, there has been a data explosion with tons of data in the form of sensor data, social media data, CRM data, web data, clinical & system data, and many more. This massive amount of data goes through an evaluation to predict behaviors and events, quality control, cost optimization, productivity calculation, etc. But what comes as a challenge here is the prediction of “something”. And this is an anomaly, anomaly is nothing but an unexpected behavior or a change in any process or system due to some internal events. This information about unexpected or unusual behavior is called an Anomaly.
Anomaly detection makes predictive maintenance effective. It is a method that determines any unusual pattern or activity that could result in system failure. For example, a mechanical system contains temperature, vibration, and speed sensors. In case the gearbox is about to fail, these sensors would provide a threshold value that can alert about the gearbox breakdown. But, with anomaly detection, you can make use of the data from available sensors and decide for an inspection even before the threshold time. This would help in taking measures to prevent gearbox failure.
You may also like reading – What is IIOT
How machine learning, and IoT app development a game changers in predictive maintenance
With machine learning and IoT app development making their way through all areas of industries. Moreover, predictive maintenance is not left behind. For industries, unnecessary downtime is the biggest issue. Hence these industries realize the importance of identifying the potential failures, their occurrence, and their consequences. To cater to this problem, organizations now use machine learning for faster and smarter data-driven decisions.
Machine learning has been in place for quite some time now, but its capacity to utilize artificial intelligence with big data in industrial infrastructure is now advancing rapidly.
Companies have been using data from visual inspections, instrument inspections, and existing condition monitoring to perform maintenance. However, with machine learning, it becomes easy to identify patterns in available data and predict machine outcomes. This is predictive analytics. It works by identifying the correct data set and combining it with machines to feed real-time data and improving data quality by tracking machines for failures. For accurate predictive maintenance, data quality is of prime importance.
For predictive maintenance, IoT app development plays a crucial role. Machine learning algorithms fetch the data from sensors that integrate with machines. These algorithms link sensors to huge amounts of data in real-time. With the use of this data, sensors can monitor equipment conditions and predict any anomalies that could lead to failure.
With Predictive maintenance, manufacturers can lower maintenance costs, lessen downtime, and extend equipment life, thereby enhancing the quality of production by attending to problems before equipment fails. However, to utilize these benefits, a flexible digital system must be set up. Moreover, includes an IoT platform such as Microsoft Azure IoT Suite and statistical methods such as predictive modeling and machine learning to analyze machine data.
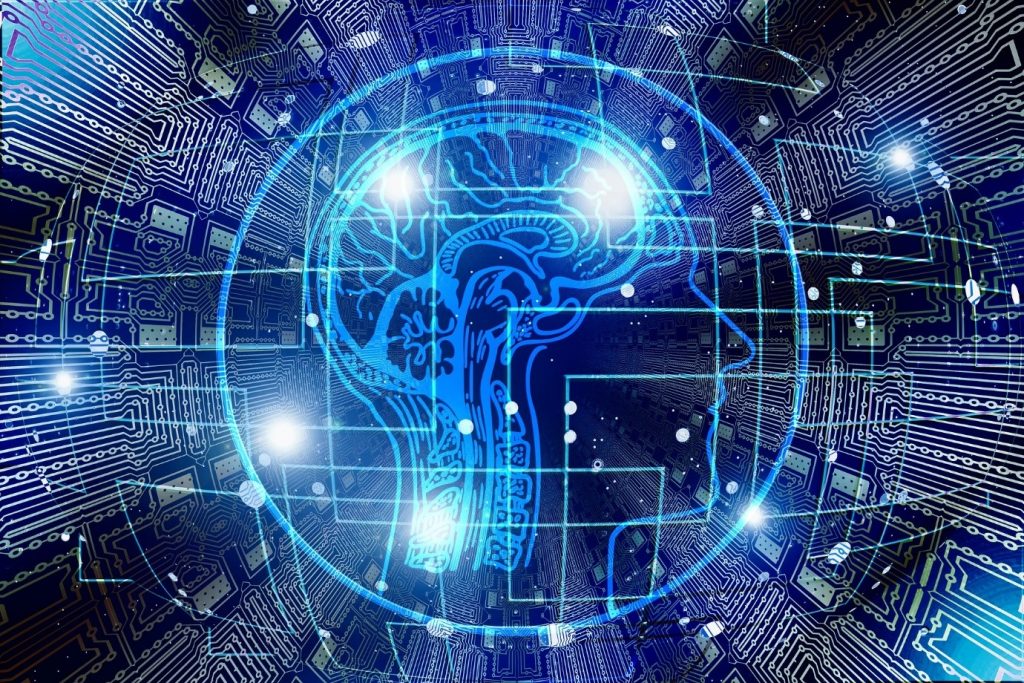
The Way Forward: Predictive Maintenance 4.0 is the future
The Internet 4.0 wave has positively hit the predictive maintenance area of the industrial domain. Machine learning combined and embedded with IoT application development and AI applications will assist organizations in managing, monitoring, and maintaining the condition of their equipment. By deploying these machine learning and AI-enabled smart solutions, companies can reduce the need for manual checks, and save cost and ample amount of time.
Sensors embedded with machine learning technology can deliver useful decision-making insights for the staff to predict machine failure and they can act fast before it crushes down.
Preventive Maintenance 4.0, along with IoT app development, is also instrumental in managing Key Performance Indicators at an industrial unit, ensuring effective health and safety measures. By monitoring and analyzing the data flow from connected equipment and manpower, potential faults can be identified and addressed proactively, helping to prevent injuries and minimize downtime.
The smart collaboration of machine learning and big data analytics has improved predictive maintenance decisions through its faster, more intelligent, and more responsive models.