Highlights on AI Predictive Analytics
- The article explores industry applications of AI predictive analytics across diverse sectors like healthcare, retail, finance, manufacturing, and more.
- It highlights how AI predictive analytics platforms work, benefits, and leverage machine learning and data analysis techniques to drive efficiency and enhance decision-making.
- Ethical considerations, compliance challenges, and data privacy concerns are addressed, underlining the importance of responsible AI practices.
- Cost and ROI of AI predictive analytics
- The discussion extends to the future of AI predictive analytics, emphasizing its potential for innovation and strategic planning.
Overview
You must have come across the statement, "Data is the new gold." Ever wondered why it was said so, and how this non-physical asset can be valued so highly that it’s compared to gold? Let's explore the details. In today’s digital age, data is incredibly valuable because it holds the key to insights that drive decision-making, innovation, and competitive advantage.
Ever noticed how online retailers like Amazon seem to know exactly what you’re looking for? Have you wondered how they manage to recommend products that are just right for you? Imagine a large hospital that deals with thousands of patients each day. Have you wondered how they manage to predict patient admissions and optimize their resources so effectively?
Today, businesses leverage data to understand customer behavior, optimize operations, predict trends, and personalize experiences, making it a critical asset for growth and efficiency. The more accurate and comprehensive the data, the better an organization can anticipate market needs, mitigate risks, and enhance profitability. Just as gold has historically been a cornerstone of wealth, data now underpins the modern economy, driving advancements in technology, healthcare, finance, and beyond. Hence, in a world increasingly driven by information, data truly is the new gold.
So, if data is indeed considered the new gold, then unlocking its value through measurement and analysis becomes paramount, doesn't it? Enter data analytics, the key that opens the door to invaluable insights hidden within the vast sea of data.
Here comes data analytics and more...
Data analytics plays a crucial role in transforming raw data into meaningful insights that drive decision-making across various industries. By analyzing historical and real-time data, organizations can gain a deeper understanding of patterns and trends.
But the power of data doesn't stop there.
Enter predictive analytics, a sophisticated branch of data analytics that not only uncovers insights from the past but also forecasts future events and behaviors, enabling businesses to anticipate and proactively respond to upcoming challenges and opportunities.
Let's explore more on this.
Predictive Analytics and Its Importance in Modern Business
Predictive analytics is revolutionizing the way businesses operate by providing insights into future events based on historical data. Moreover, this advanced analytical technique helps companies anticipate trends, optimize operations, and make informed decisions. In today's competitive landscape, leveraging predictive analytics is crucial for maintaining a competitive edge. In fact, businesses can proactively address challenges and seize opportunities by analyzing patterns and predicting outcomes.
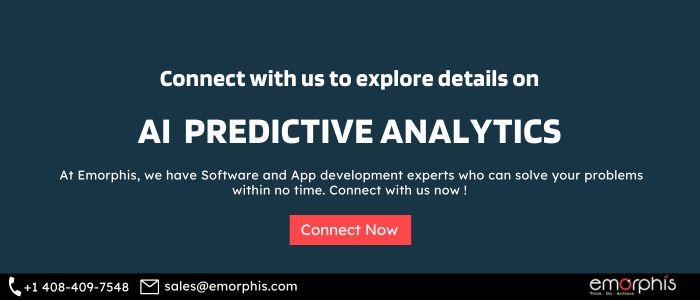
Understanding Predictive Analytics - Definition and Key Concepts
Predictive analytics involves using statistical techniques, data mining, and machine learning to analyze historical data moreover, predict future outcomes. By identifying patterns and trends, businesses can forecast events such as customer behavior, market trends, and operational performance.
The foundation of predictive analytics is historical data. Machine learning techniques analyze this data to uncover relationships and patterns. Moreover, algorithms learn from past data to make predictions about future events. Also, common methods include regression analysis, classification, and clustering.
Big data technology can be highly inclusive in driving predictive analytics.
Moreover, with the increasing volume of data generated by businesses, having the ability to process and analyze large datasets is essential. Big data provides the raw material that machine learning algorithms need to make accurate predictions.
AI Technologies Enhancing Predictive Analytics – Key Features of AI Predictive Analytics
Machine Learning
Machine learning is a core component of AI predictive analytics. It involves training algorithms to recognize patterns in data and also make predictions. Moreover, the benefits of machine learning include improved accuracy, the ability to handle large datasets, and continuous learning from new data. This makes it invaluable for tasks such as fraud detection, customer segmentation, and demand forecasting.
Deep Learning
Deep learning, a subset of machine learning, uses neural networks with multiple layers to analyze complex data. This technology is particularly useful for tasks that require high levels of abstraction, such as image and speech recognition. In predictive analytics, deep learning can also enhance the accuracy of models by capturing intricate patterns in the data.
Natural Language Processing (NLP)
Natural Language Processing (NLP) allows AI systems to understand and moreover, interpret human language. This technology is essential for analyzing text data, such as customer reviews and social media posts. NLP can provide insights into customer sentiment, identify emerging trends, also, enhance customer service through chatbots and virtual assistants.
Computer Vision - Visual Data Interpretation and Applications
Computer vision enables machines to interpret visual data from images and videos. In predictive analytics, this technology can be used for tasks such as monitoring consumer behavior in retail stores or identifying potential equipment failures in manufacturing. Moreover, businesses can gain valuable insights and improve operational efficiency by analyzing visual cues.
Reinforcement Learning
Reinforcement learning involves training AI systems to make decisions by rewarding desired behaviors. Moreover, this technology is useful for optimizing complex processes that require a sequence of decisions. In fact, in logistics and supply chain management, reinforcement learning can optimize routes and inventory levels, leading to cost savings and improved service delivery.
Benefits of Using AI Predictive Analytics
1. Enhanced Accuracy
AI predictive analytics provides more accurate forecasts by identifying complex patterns in large datasets that traditional methods might miss. This, in fact, results in improved decision-making and strategic planning.
2. Real-Time Analysis
AI systems can process and analyze data in real-time, offering immediate insights for crucial time-sensitive decisions. This capability is particularly valuable in industries like finance, healthcare, and retail.
3. Improved Customer Insights
AI predictive analytics can predict future behaviors and preferences, by analyzing customer data. This allows businesses to tailor their marketing strategies, improve customer engagement, and enhance overall customer experience.
4. Risk Management
AI predictive analytics helps identify potential risks by analyzing historical data. Also, forecasting future trends. Moreover, this allows businesses to take proactive measures to mitigate risks and avoid costly disruptions.
5. Operational Efficiency
Automating data analysis with AI, in fact, reduces the need for manual processing, saving time and resources. This leads to enhanced operational efficiency and cost savings.
6. Personalization
AI predictive analytics enables businesses to offer personalized experiences to customers. As a matter of fact, it is done by predicting their preferences and behaviors. This enhances customer satisfaction and loyalty.
7. Competitive Advantage
By leveraging AI predictive analytics, businesses can stay ahead of market trends. Moreover, competition, the ability to anticipate changes and adapt quickly provides a significant competitive edge.
8. Continuous Improvement
AI models can continuously learn and adapt to new data. Also, ensuring that predictions remain accurate and relevant over time. Moreover, this dynamic learning capability supports ongoing improvement and innovation.
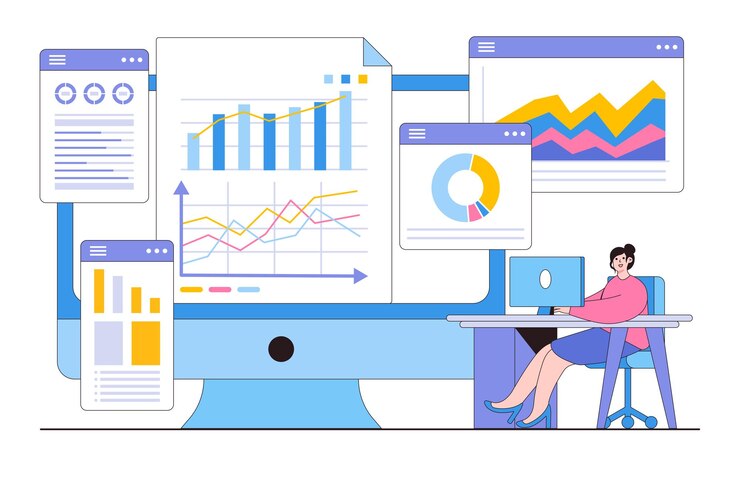
Industry Applications of AI Predictive Analytics
AI predictive analytics integrated across diverse industries further, reshape how businesses operate and make decisions. Let's explore the specific applications also, the software solutions powered by AI predictive analytics in various sectors.
Healthcare
In healthcare, the applications of AI predictive analytics are multifaceted. Also, transformative too. Software solutions utilizing AI algorithms can aid in early disease detection by analyzing patient data. Moreover, to identify subtle patterns indicative of potential health risks. Additionally, predictive analytics tools can assist in personalized treatment planning, leveraging patient-specific information to tailor interventions and optimize health outcomes. Disease outbreak prediction software integration with AI predictive analytics can help healthcare providers anticipate and mitigate public health crises. Moreover, by analyzing global health data to forecast potential epidemic hotspots. Also, diagnostic imaging software enhanced with AI algorithms offers more accurate interpretations of medical images, facilitating early detection and treatment planning for various conditions.
Find details on Healthcare Software Product Engineering and Generative AI use cases.
Retail
AI predictive analytics is revolutionizing the retail sector. It is in fact, powering a range of software solutions designed to optimize business operations and enhance customer experiences. Inventory management software utilizes predictive analytics to forecast future demand based on historical sales data also, seasonal trends, and market dynamics, enabling retailers to maintain optimal stock levels and minimize stockouts. Moreover, today Customer relationship management (CRM) software integrated with AI predictive analytics offers insights into consumer behaviors and preferences, enabling personalized marketing campaigns and targeted promotions. Dynamic pricing software adjusts prices in real-time based on demand and market conditions. As a matter of fact, maximizing profitability and competitiveness. Additionally, demand forecasting software utilizes AI algorithms to analyze complex data sets and predict future demand, enabling retailers to optimize their supply chain and inventory management strategies.
Find details of Retail and eCommerce Software Development Services
Finance and Banking
In finance and banking, AI predictive analytics drives innovation and efficiency across various functions, from risk management to customer engagement. In fact, fraud detection software powered by AI algorithms analyzes transaction patterns and detects anomalies indicative of fraudulent activity, enabling financial institutions to safeguard against financial crimes. Moreover, credit scoring software utilizes predictive analytics to assess the creditworthiness of individuals and businesses, leveraging AI algorithms to analyze traditional and non-traditional data sources. Investment management software integrated with AI predictive analytics can help investors identify market trends, in fact, it can also, assess investment opportunities, and optimize their investment strategies. Additionally, predictive analytics software aids in risk management by forecasting potential risks and identifying opportunities for mitigating financial losses.
Find more details on Fintech App Development
Manufacturing
In manufacturing, AI predictive analytics is instrumental in optimizing production processes. Thus, enhancing quality control, and improving resource efficiency. Predictive maintenance software powered by AI algorithms analyzes equipment data. In fact, it is to predict potential failures and schedule maintenance activities proactively, minimizing downtime and optimizing asset performance. Quality control software utilizes AI predictive analytics to moreover, detect defects and anomalies in manufacturing processes, ensuring product quality and reducing waste. Resource optimization software leverages predictive analytics to optimize resource allocation. Also, it helps minimize production costs and improve operational efficiency. Additionally, predictive analytics software aids in demand forecasting, enabling manufacturers to anticipate market trends and adjust production schedules accordingly.
Find details on Industrial Software Development
Startups
Startups are increasingly leveraging AI predictive analytics. As a matter of fact, to gain a competitive edge and drive innovation across various sectors. Customer acquisition software powered by AI algorithms analyzes consumer data to identify target demographics. Also, personalize marketing campaigns, and optimize customer acquisition strategies. Product development software utilizes predictive analytics to forecast market demand. Moreover, to identify product opportunities, and prioritize feature development. Operational efficiency software integrates AI predictive analytics to streamline business processes. In fact, to automate repetitive tasks, and improve resource allocation. Additionally, risk assessment software aids startups in identifying potential risks, mitigating financial losses, and ensuring long-term sustainability.
Find more details on Software Development for Startups
Services Industry
In the services industry, AI predictive analytics transforms customer engagement, operational efficiency, and service delivery. Moreover, Customer relationship management (CRM) software integrated with AI algorithms analyzes customer data to personalize interactions, anticipate needs, and enhance customer satisfaction. Predictive analytics software aids in workforce management by forecasting staffing requirements, optimizing schedules, also, improving employee productivity. Service optimization software utilizes predictive analytics to maximize service delivery processes, and minimize service disruptions. Moreover, improve service quality. Additionally, predictive analytics software aids in revenue forecasting, enabling service providers to anticipate market trends, identify growth opportunities, and optimize pricing strategies.
Find more details on Software Product Development
Agriculture
In agriculture, AI predictive analytics is revolutionizing crop management, yield prediction, also, resource optimization. Crop management software powered by AI algorithms analyzes environmental data, soil conditions, also, crop health metrics to optimize planting schedules, predict crop yields, and mitigate risks. Yield prediction software utilizes predictive analytics to forecast crop yields based on historical data, also weather forecasts, and agronomic factors, enabling farmers to make informed decisions and optimize harvest operations. Resource optimization software integrates AI predictive analytics to optimize water usage, fertilizer application, and pesticide application, maximizing agricultural productivity while minimizing environmental impact. Additionally, market analysis software aids farmers in identifying market trends, assessing demand, and optimizing crop selection to maximize profitability.
Find details of Smart Farming
Real Estate
In the real estate sector, AI predictive analytics is driving innovation in property valuation, market analysis, and investment decision-making. Property valuation software powered by AI algorithms analyzes property data, market trends, and comparable sales to accurately estimate property values, enabling real estate professionals to make informed pricing decisions. Market analysis software utilizes predictive analytics to forecast market trends, identify emerging opportunities, and assess investment risks, empowering investors to make strategic decisions. Investment analysis software integrates AI predictive analytics to evaluate investment opportunities, analyze potential returns, and optimize investment portfolios. Additionally, predictive analytics software aids in property management by predicting rental demand, optimizing rental pricing, and identifying maintenance needs to maximize rental income and property value.
Other Trending Industries
In addition to the sectors, AI predictive analytics is making significant strides in other trending industries, such as transportation, education, and environmental sustainability. Transportation optimization software powered by AI algorithms analyzes traffic data, route information, and vehicle telemetry to optimize transportation routes, reduce congestion, and improve fuel efficiency. Education analytics software utilizes predictive analytics to forecast student performance, identify at-risk students, and personalize learning experiences to improve educational outcomes. Environmental sustainability software integrates AI predictive analytics to monitor environmental data, predict climate change impacts, and optimize resource management practices to promote sustainability and mitigate environmental risks. Additionally, predictive analytics software aids in trend analysis, market forecasting, and strategic planning across various emerging industries, empowering businesses to stay ahead of the curve and capitalize on new opportunities.
Overall, AI predictive analytics is a transformative technology that is revolutionizing business operations across diverse industries. By leveraging AI algorithms to analyze data, predict trends, and make informed decisions, organizations can gain actionable insights, drive innovation, and achieve sustainable growth in today's dynamic marketplace. As AI technology continues to evolve, its potential to drive innovation and create new opportunities across industries is limitless, making it an indispensable tool for businesses seeking to thrive in the digital age.
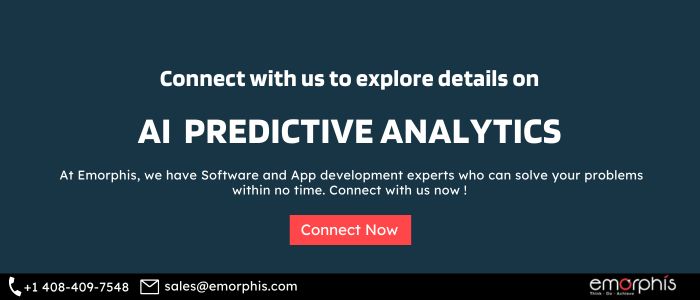
How Does AI Predictive Analytics Platform Work?
AI predictive analytics platforms are sophisticated systems that execute a series of intricate processes to unlock actionable insights and predict future outcomes with precision. Let's explore each step in greater detail:
1. Data Collection and Preprocessing
- Data Sourcing - Gathering vast volumes of data from various sources including transaction records, customer interactions, social media, and IoT devices.
- Now, Data Cleansing - Scrutinizing collected data to identify and rectify inaccuracies, inconsistencies, and missing values.
- Data Transformation - Normalizing and structuring the data to ensure uniformity and compatibility for analysis.
- Feature Engineering - Selecting and extracting relevant features from the data that hold predictive value for modeling.
2. Model Training and Development
- Algorithm Selection - Choosing appropriate machine learning algorithms based on the nature of the problem and the characteristics of the data.
- Training Data Preparation - Dividing the data into training and validation sets to train the models while assessing their performance.
- Model Training - Utilizing machine learning algorithms to train predictive models on historical data, enabling them to learn patterns and relationships.
- Hyperparameter Tuning - Optimizing model performance by fine-tuning hyperparameters such as learning rates and regularization terms.
3. Predictive Analysis and Insights Generation
- Data Analysis - Deploying trained models to analyze new data inputs and generate predictions.
- Insight Generation - Deriving actionable insights from the predictions to inform decision-making processes.
- Visualization - Presenting the insights in visually appealing formats such as charts, graphs, and dashboards for better comprehension.
4. Continuous Learning and Optimization
- Feedback Loop Integration - Incorporating feedback mechanisms to continuously learn from new data and refine predictive models.
- Model Evaluation - Assessing model performance regularly using metrics such as accuracy, precision, recall, and F1-score.
- Model Updating - Updating and retraining models periodically to adapt to changing data patterns and evolving business requirements.
- Ensemble Learning - Employing ensemble learning techniques to combine multiple models for enhanced predictive accuracy and robustness.
By meticulously executing these processes, AI predictive analytics platforms can transform raw data into valuable insights, empowering organizations to make data-driven decisions, mitigate risks, and seize opportunities in an ever-evolving landscape.
Impact, Challenges, and Opportunities of Ethical Considerations, Compliance, and Data Privacy in AI Predictive Analytics
Impact
- Trust and Transparency - Addressing ethical considerations, compliance, and data privacy concerns enhances trust and transparency in AI predictive analytics systems. Stakeholders feel more confident in the integrity of the data and the decisions derived from it.
- Reputation Management - Adhering to ethical standards and data privacy regulations safeguards organizations' reputations. It demonstrates their commitment to responsible data practices, fostering positive relationships with customers, partners, and regulators.
- Risk Mitigation - By prioritizing ethical considerations and data privacy, organizations mitigate the risk of data breaches, regulatory penalties, and legal liabilities. Proactive measures protect sensitive information and minimize potential harm to individuals.
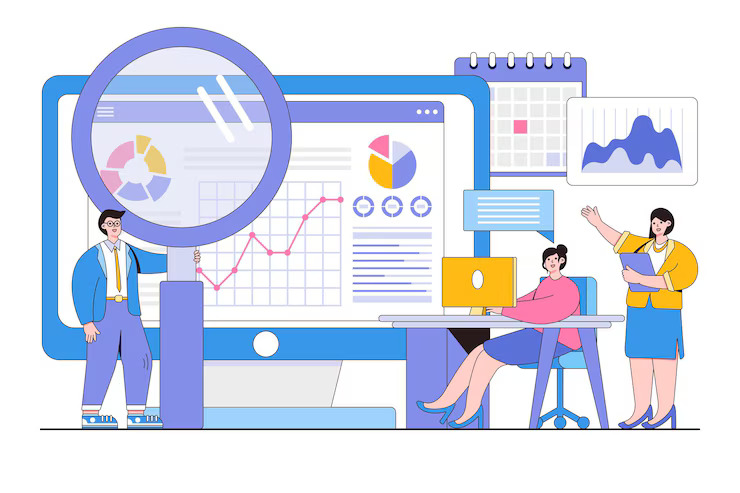
Challenges
- Data Quality and Bias - Ensuring data quality and mitigating bias in AI models pose significant challenges. Biased or inaccurate data can lead to unfair outcomes and perpetuate existing inequalities, undermining the ethical integrity of predictive analytics.
- Regulatory Compliance - Navigating complex and evolving regulatory landscapes, such as GDPR in Europe or CCPA in California, requires dedicated resources and expertise. Compliance with diverse regulations across jurisdictions adds layers of complexity to AI deployments.
- Algorithmic Transparency - Achieving algorithmic transparency remains a challenge, particularly with complex machine learning models. Understanding how AI systems arrive at decisions is crucial for accountability and trust, yet it can be difficult to interpret opaque algorithms.
Opportunities
- Innovation in Ethical AI - Embracing ethical considerations fosters innovation in AI development. It encourages the creation of tools and frameworks for responsible AI, such as explainable AI (XAI) techniques and fairness-aware algorithms.
- Competitive Advantage - Organizations that prioritize ethical AI and data privacy gain a competitive advantage. Ethical data practices differentiate them in the marketplace, attracting customers who value privacy and transparency.
- Collaborative Ecosystems - Collaboration across industries and stakeholders drives progress in ethical AI. By sharing best practices, tools, and resources, organizations can collectively address challenges and promote responsible data use.
While ethical considerations, compliance, and data privacy present challenges for analytics, they also offer opportunities for innovation, trust-building, and competitive differentiation. By prioritizing ethical principles and responsible data practices, organizations can harness the full potential of AI while safeguarding individual rights and societal values.
Cost, ROI of AI Predictive Analytics
The cost of developing and integrating AI predictive analytics solutions can vary depending on factors such as the complexity of the project, the specific industry requirements, and the sophistication of the AI algorithms involved. Initial development costs may include expenses related to data collection, preprocessing, algorithm development, and software integration. Additionally, ongoing costs for maintenance, updates, and data management should be considered.
Despite the initial investment, the return on investment (ROI) of AI predictive analytics can be substantial. By enabling more informed decision-making, optimizing operations, reducing risks, and uncovering new opportunities, It can deliver tangible benefits such as increased revenue, cost savings, improved efficiency, and enhanced customer satisfaction. Over time, the ROI of AI predictive analytics is likely to outweigh the initial investment, making it an asset for businesses seeking to gain a competitive edge in today's data-driven landscape.
What is the Future of AI Predictive Analytics?
As we navigate the rapidly evolving landscape of technology, the future of AI predictive analytics holds immense promise and potential. Let's peer into what lies ahead.
a. Enhanced Accuracy and Precision
- With advancements in machine learning algorithms and deep learning techniques, AI predictive analytics will offer even greater accuracy and precision in forecasting future trends and outcomes.
- Improved models will be better equipped to handle complex and high-dimensional data, leading to more nuanced predictions and actionable insights.
b. Real-time Predictions
- The future of AI predictive analytics will witness a shift towards real-time predictive capabilities, enabling organizations to make instant decisions based on up-to-the-minute data.
- Rapid processing speeds and advancements in streaming analytics will facilitate timely insights, empowering businesses to respond swiftly to changing market dynamics and customer behaviors.
c. Explainable AI
- As AI continues to permeate various aspects of decision-making, there will be a growing emphasis on explainable AI, where predictive models provide transparent explanations for their predictions.
- Interpretability and transparency will become essential features of AI predictive analytics systems, fostering trust and confidence among users and stakeholders.
d. AI-driven Automation
- The integration of AI predictive analytics with automation technologies will revolutionize business operations, streamlining processes, and optimizing resource allocation.
- Automated decision-making based on predictive insights will become commonplace across industries, driving efficiency and productivity to new heights.
e. Personalization at Scale
- AI predictive analytics will enable hyper-personalization at scale, allowing businesses to tailor products, services, and experiences to individual preferences and behaviors.
- Advanced segmentation techniques and predictive modeling will facilitate targeted marketing campaigns, customized recommendations, and personalized customer interactions.
f. Ethical Considerations and Bias Mitigation
- The future of analytics will place a greater emphasis on ethical considerations and bias mitigation strategies.
- Efforts to ensure fairness, transparency, and accountability in predictive modeling will become paramount, addressing concerns related to data bias, privacy, and algorithmic discrimination.
g. Cross-Industry Applications
- AI predictive analytics will continue to transcend traditional boundaries, finding applications across diverse industries including healthcare, finance, retail, manufacturing, and beyond.
- Cross-industry collaborations and knowledge sharing will fuel innovation, driving the development of new predictive models and applications.
The future of AI predictive analytics is poised to revolutionize decision-making, drive innovation, and unlock new opportunities for businesses and organizations worldwide. By harnessing the power of data and AI technologies, we embark on a journey toward a future where foresight is not just a luxury but a strategic imperative for success.
Why Choose Emorphis Technologies For AI Predictive Analytics Solutions?
Emorphis Technologies offers comprehensive expertise and solutions to aid organizations in developing and integrating AI predictive analytics seamlessly into their operations. Leveraging advanced AI technologies and a wealth of experience, Emorphis Technologies can tailor predictive analytics solutions to meet specific organizational needs across various industries. Their team of skilled professionals guides clients through every step of the development process, from data collection and preprocessing to algorithm development and integration with existing systems.
Steps to Implement AI Predictive Analytics
- Identify Business Needs: Assess where AI predictive analytics can benefit your organization the most and define clear objectives for implementation.
- Collaborate with Emorphis: Work closely with Emorphis Technologies to communicate your requirements, goals, and expectations for the project.
- Develop a Plan: Create a detailed roadmap with Emorphis Technologies outlining the steps involved in developing and integrating the solution.
- Customize Solutions: Emorphis Technologies will tailor analytics solutions to fit your specific business needs, leveraging advanced AI technologies.
- Seamless Integration: Emorphis Technologies will assist in integrating the solution seamlessly into your existing infrastructure to minimize disruption.
- Ongoing Support: Emorphis Technologies provides continuous support, monitoring, and optimization to ensure the effectiveness and performance of the predictive analytics solution.
Once the predictive analytics solution is developed, Emorphis Technologies assists in the seamless integration of the solution into existing infrastructure, ensuring minimal disruption to operations. Post-implementation, Emorphis Technologies provides ongoing support, monitoring, and optimization to maximize the effectiveness and performance of the predictive analytics solution.
By partnering with Emorphis Technologies, organizations can unlock the full potential of AI predictive analytics to drive informed decision-making, optimize processes, and achieve their business objectives effectively.
Conclusion
In summary, the article offers a comprehensive overview of AI predictive analytics, examining its industry applications, operational mechanisms, prospects, and ethical implications. It underscores the transformative impact of AI predictive analytics across diverse sectors while emphasizing the need for ethical and responsible AI deployment.